As the demand for more intelligent and context-aware AI grows, Vision-Language Models (VLMs) have emerged as a powerful class of models capable of understanding both images and text. These models power applications such as AI assistants, medical document analysis, and automated insurance claim processing.
This article provides practical, experience-based best practices for training large VLMs using Metal Cloud, offering a scalable and high-performance AI infrastructure fueled by NVIDIA H100 GPUs. Whether you're an AI engineer, data scientist, or IT decision-maker looking to scale multimodal AI systems efficiently, this guide walks you through the architectural choices, training pipelines, and optimization strategies proven to deliver real-world impact.
1. Real-World Applications and Deployment Outcomes
VLMs are transforming multiple industries:
- Document Understanding & Intelligent Document Processing (IDP): Extracting insights from unstructured formats and images.
- Medical & Insurance Analysis: Automating claims processing, including data entry and the adjustment process, detecting fraudulent claims, and summarizing medical documents.
- Example of medical documents:
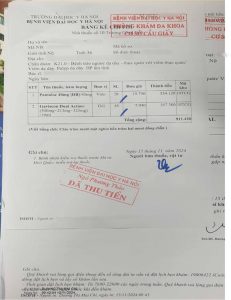
- AI-Powered Assistants: Enabling AI chatbots with multimodal reasoning and contextual awareness.
- Business Impact of PDF Data Extraction:
- Reduced manual data entry time from 15 minutes to under 2 minutes.
- Faster adaptation to new datasets, reducing training duration from months to weeks.
- Scaled processing capacity without increasing reliance on human resource
- Enhanced fraud detection capabilities through AI-driven analysis.
- Based on the NVIDIA VSS Blueprint Architecture, FPT AI Factory has implemented it in automated vehicle insurance claim video processing, using the following architecture:
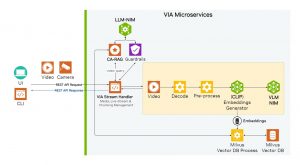
Figure. High-level architecture of the summarization vision AI agent
- Business Impact of accessing car information and damage assessments:
- Automated Damage Evaluation: Use VLM to analyze claim descriptions and video for automated damage assessment. VLM model categorizes cases into severe damage, minor damage, or no damage, directing them to the appropriate processing streams and experts. This approach enables automation of up to 80% of minor damage claims, reducing claim processing time from 20 minutes to just 2 minutes.
- Enhancing Claims Processing Efficiency: Minimize human intervention and expedite claim settlements through AI-powered assessments
- Detecting and Preventing Fraud: Identify anomalies and inconsistencies in claim reports to mitigate fraud
- Optimizing Operational Costs: Reduce expenses associated with manual inspections and assessment processes
- Example of car damage assessment with VLM
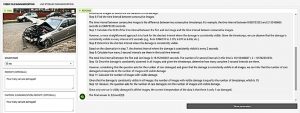
ROI of H100 Over A100
- Higher initial cost, but lower total expenditure due to efficiency.
- Shorter training cycles, leading to faster model deployment.
- Estimated 43% reduction in overall training cost compared to A100.
2. VLM Architecture, Data Processing Pipeline, and Hardware Requirements
2.1 VLM Architecture
A standard VLM consists of three key components:
- Vision Encoder: Utilize CNN or transformer-based models such as ViT, CLIP Vision Encoder, and Swin Transformer to extract image features.
- Language Decoder: Implement LLMs such as GPT, LLaMA, and Qwen to generate textual outputs based on visual prompts.
- Multimodal Fusion Module: Integrate image and text embeddings for cohesive output generation.
2.2 Data Processing Pipeline
The pipeline for processing image and text data in VLM training follows these key steps:
- Image data is passed through the Vision Encoder, extracting relevant visual features.
- Text data is processed using a Text Embedder, converting it into vector representations.
- Both vision and text embeddings are then fused and passed into the Language Model with Self-Attention Layers, enabling multimodal learning.
- Zero-shot Visual Question Answering (VQA): The trained model can answer questions about new images it has never encoutered before.
- Few-shot Image Classification: By leveraging learned embeddings, the model can classify new images with minimal labeled examples.
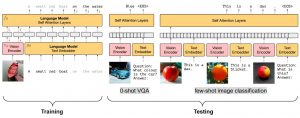
2.3 NVIDIA software stack in use
Training:
We use NVIDIA NeMo for our fine-tuning task. NVIDIA NeMo is an open-source framework designed for training and fine-tuning large-scale AI models, including vision-language models (VLMs), speech models, and NLP models.
The NVIDIA Nemo framework supports many utilities:
- Pretrained Foundation Models: Providing optimized foundation models that can be fine-tuned for specific applications.
- Model Parallelism: Supporting tensor, pipeline, and sequence parallelism, enabling the training of extremely large-scale models on multiple GPUs or nodes.
- LoRA and QLoRA Support: Reducing compute and memory costs while maintaining accuracy through efficient parameter-efficient fine-tuning methods.
- Integration with NVIDIA HGX Cloud: Enabling seamless cloud-based training on clusters powered by H100 GPUs.
Performance Gains with NVIDIA NeMo on H100 GPUs
✅ 2-3x Faster Training with FP8 precision and optimized kernels
✅ 50% Lower Memory Usage using mixed precision and memory-efficient optimizers
✅ Seamless Multi-GPU Scaling with Tensor & Pipeline Parallelism
By leveraging NVIDIA NeMo on H100 GPUs, we can fine-tune VLMs efficiently at scale, reducing both compute cost and time to deployment.
Inferencing:
In order to maximize the performance of the VLM with the low latency but high throughput requirements, we utilize the TensorRL-LLM as an optimizer for VLM. With TensorRT-LLM, we achieve significantly lower latency overall and also lower TTFT. TensorRT-LLM also supports a wide range of quantization, including INT8, SmoothQuant, FPT8, INT4, GPTQ, and AWQ.
2.4 Hardware Considerations
For effective training, key hardware factors include:
- Batch Size and Sequence Length: Optimized for maximum GPU utilization without memory bottlenecks.
- Memory Management: Leveraging H100’s high-bandwidth memory for efficient data processing.
- Parallelization Strategies: Using tensor parallelism, pipeline parallelism, and distributed training techniques to optimize large-scale models.
3. Benchmarking NVIDIA H100 vs. A100 GPUs for VLM Training
While the NVIDIA H100 GPU has a higher hourly operational cost than the A100, it significantly reduces overall training expenses due to shorter training times. Case studies indicate that training on the H100 reduces costs by approximately 43% and accelerates training by a factor of 3.5x compared to A100.
Performance comparisons highlight H100’s superior efficiency:
Metric |
2 x H100 (HBM3-80GB) |
2 x A100 (PCIe-80GB) |
Higher is Better? |
Epoch Time (Qwen2.5VL-7B, batch_size=2, num_sample=200k) |
~24 h |
~84 h |
No |
Inference Throughput (Qwen2.5VL-3B, token/sec, PyTorch) |
~410 |
~150 |
Yes |
Power Consumption (100% GPU utilization, per card) |
480W |
250W |
No |
Hourly Cost |
1.5 x A100 |
Lower |
No |
Total Training Cost |
0.57 x A100 |
Higher |
No |
4. Lessons Learned and Optimization Strategies
Resource Optimization
- Maximizing GPU Utilization: Proper tuning of batch size, sequence length, and caching mechanisms.
- Parallel Processing Strategies: Implementing FSDP, ZeRO, and NCCL to improve training speed.
Distributed Training Challenges
- Data Synchronization: Efficient GPU communication to avoid bottlenecks.
- Infrastructure Readiness: Ensuring power and cooling support for high-energy-consuming H100 clusters.
System Integration & Stability
- Software Stack Compatibility: Ensuring seamless operation with PyTorch/XLA, Triton, and TensorRT.
- Continuous Performance Monitoring: Regular fine-tuning to maintain optimal efficiency.
5. Future Trends in VLM Training Optimization
To address increasing model complexity and computational demands, several trends are shaping the optimization of VLM training:
- Scalability and Efficiency: FP8 precision, quantization techniques, and FlashAttention optimize memory utilization, ensuring fast processing.
- Advanced Training Pipelines: Techniques like ZeRO (DeepSpeed) and Fully Sharded Data Parallel (FSDP) reduce memory overhead and improve scalability.
- High-Performance Multi-GPU Training: H100’s NVLink 4.0 and PCIe 5.0 enable faster inter-GPU communication, minimizing bottlenecks.
- Efficient Fine-Tuning Techniques: Methods such as LoRA and QLoRA allow efficient parameter tuning while reducing computational costs.
- Domain-Specific Optimization: Future VLMs will be fine-tuned for specialized domains like medical imaging, legal document processing, and technical analysis, requiring tailored datasets and optimized training strategies.
6. Conclusion & Recommendations
When to Choose H100
- Training large-scale VLMs (7B+ parameters) requiring high batch sizes and long sequence lengths.
- Deploying multi-GPU clusters with NVLink 4.0 for enhanced interconnect speeds.
- Use cases demanding real-time inference with minimal latency.
When A100 is Sufficient
- Smaller-scale GenAI models (under 4B parameters) with relaxed training time constraints.
- Cost-sensitive projects where training duration is less critical.
- Single-task models requiring less computational complexity.
Final Thoughts
With increasing demands for more sophisticated VLMs, optimizing hardware, algorithms, and training strategies remains essential. The NVIDIA H100 GPUs stands out as the preferred choice for large-scale, high-performance VLM training, driving advancements in multimodal AI and accelerating real-world applications.
Learn more about FPT AI Factory's services HERE.
For more information and consultancy about FPT AI Factory, please contact: